In our new manuscript we developed machine learning-based models to predict the toxicity of binary pesticide mixtures in honey bees
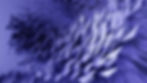
Our research, just published in the prestigious Journal of Hazardous Materials, used quantitative read-across structure-activity relationship (q-RASAR) modelling to predict the binary mixture toxicity of untested molecules via acute contact mode of actions.
We implemented recently published toxicity data on honey bees and used both quantitative structure-activity relationship (QSAR) and similarity-based read-across algorithms to simultaneously enhance the predictability of the model. Machine learning algorithms like ridge regression, linear support vector machine (SVM), and non-linear SVM have been used to further enhance the predictability of the q-RASAR model. The prediction quality of the q-RASAR models outperforms previous models. We have predicted the toxicity of several new untested binary mixtures, validating results' reliability.
We produced a reliable, complementary, and integrative q-RASAR modelling approach that outperforms conventional experimental approaches when predicting the toxicity of pesticide mixtures in risk assessment processes.
Chatterjee M, Banerjee A, Tosi S, Carnesecchi E, Benfenati E, Roy K (2023) Machine learning - based q-RASAR modeling to predict acute contact toxicity of binary organic pesticide mixtures in honey bees. J. Hazard. Mater. 460, 132358 https://doi.org/10.1016/j.jhazmat.2023.132358
Tosi, S., Sfeir, C., Carnesecchi, E., VanEngelsdorp, D., Chauzat, M.-P., 2022. Lethal, sublethal, and combined effects of pesticides on bees: A meta-analysis and new risk assessment tools. Sci. Total Environ. 844, 156857. https://doi.org/10.1016/j.scitotenv.2022.156857